Por favor, use este identificador para citar o enlazar este ítem:
http://hdl.handle.net/20.500.11765/14650
Improving global hydrological simulations through bias-correction and multi-model blending
Título : | Improving global hydrological simulations through bias-correction and multi-model blending |
Autor : | Chevuturi, Amulya; Tanguy, Maliko; Facer-Childs, Katie; Martínez de la Torre, Alberto![]() |
Palabras clave : | Global hydrological forecasts; Hydrological models; ULYSSES; Bias-correction; HydroSOS; Multi-model blending |
Fecha de publicación : | 2023 |
Editor: | Elsevier |
Citación : | Journal of Hydrology. 2023, 621, 129607 |
Versión del editor: | https://doi.org/10.1016/j.jhydrol.2023.129607 |
Resumen : | There is an immediate need to develop accurate and reliable global hydrological forecasts in light of the future vulnerability to hydrological hazards and water scarcity under a changing climate. As a part of the World Meteorological Organization’s (WMO) Global Hydrological Status and Outlook System (HydroSOS) initiative, we investigated different approaches to blending multi-model simulations for developing holistic operational global forecasts. The ULYSSES (mULti-model hYdrological SeaSonal prEdictionS system) dataset, to be published as ‘‘Global seasonal forecasts and reforecasts of river discharge and related hydrological variables ensemble from four state-of-the-art land surface and hydrological models’’ is used in this study. The first step for improving these forecasts is to investigate ways to improve the model simulations, as global models are not calibrated for local conditions. The analysis was performed over 119 different catchments worldwide for the baseline period of 1981–2019 for three variables: evapotranspiration, surface soil moisture and streamflow. This study evaluated blending approaches with a performance metric based (weighted) averaging of the multi-model simulations, using the catchment’s Kling-Gupta Efficiency (KGE) for the variable to define the weight. Hydrological model simulations were also bias-corrected to improve the multi-model blending output. Weighted blending in conjunction with bias-correction provided the best improvement in performance for the catchments investigated. Applying modelled weights during blending original simulations improved performance over ungauged catchments. The results indicate that there is potential to successfully and easily implement the bias-corrected weighted blending approach to improve operational forecasts globally. This work can be used to improve water resources management and hydrological hazard mitigation, especially in data-sparse regions. |
Patrocinador: | This research was funded by the Natural Environment Research Council, UK through UKCEH’s SUNRISE (award number NE/R000131/1) and NC-International (award number NE/X006247/1) programmes delivering National Capability, as well as the Net Zero Capacity Building Project and UKRI-NERC STF Global Water Tools Project. |
URI : | http://hdl.handle.net/20.500.11765/14650 |
ISSN : | 0022-1694 |
Colecciones: | Artículos científicos 2023-2026 |
Ficheros en este ítem:
Fichero | Descripción | Tamaño | Formato | ||
---|---|---|---|---|---|
![]() | Supplementar.pdf | 10,63 MB | Adobe PDF | ![]() Visualizar/Abrir | |
![]() | JH_Chevuturi_2023.pdf | 2,73 MB | Adobe PDF | ![]() Visualizar/Abrir |
Los ítems de Arcimis están protegidos por una Licencia Creative Commons, salvo que se indique lo contrario.
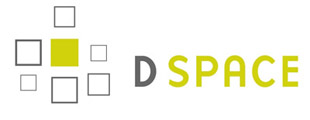