Por favor, use este identificador para citar o enlazar este ítem:
http://hdl.handle.net/20.500.11765/15287
On the limitations of deep learning for statistical downscaling of climate change projections : the transferability and the extrapolation issues
Registro completo de metadatos
Campo DC | Valor | Lengua/Idioma |
---|---|---|
dc.contributor.author | Hernanz Lázaro, Alfonso | es_ES |
dc.contributor.author | Correa, Carlos | es_ES |
dc.contributor.author | Sánchez Perrino, Juan Carlos | es_ES |
dc.contributor.author | Prieto-Rico, Ignacio | es_ES |
dc.contributor.author | Rodríguez Guisado, Esteban | es_ES |
dc.contributor.author | Domínguez Alonso, Marta | es_ES |
dc.contributor.author | Rodríguez Camino, Ernesto | es_ES |
dc.date.accessioned | 2023-12-14T08:33:55Z | - |
dc.date.available | 2023-12-14T08:33:55Z | - |
dc.date.issued | 2023 | - |
dc.identifier.citation | Atmospheric Science Letters. 2023, e1195 | es_ES |
dc.identifier.issn | 1530-261X | - |
dc.identifier.uri | http://hdl.handle.net/20.500.11765/15287 | - |
dc.description.abstract | Convolutional neural networks (CNNs) have become one of the state-of-the-art techniques for downscaling climate projections. They are being applied under Perfect-Prognosis (trained in a historical period with observations) and hybrid approaches (as Regional Climate Models (RCMs) emulators), with satisfactory results. Nevertheless, two important aspects have not been, to our knowledge, properly assessed yet: (1) their performance as emulators for other Earth System Models (ESMs) different to the one used for training, and (2) their performance under extrapolation, that is, when applied outside of their calibration range. In this study, we use UNET, a popular CNN, to assess these two aspects through two pseudo-reality experiments, and we compare it with simpler emulators: an interpolation and a linear regression. The RCA4 regional model, with 0.11° resolution over a complex domain centered in the Pyrenees, and driven by the CNRM-CM5 global model is used to train the emulators. Two frameworks are followed for the training: predictors are taken (1) from the upscaled RCM and (2) from the ESM. In both frameworks, the performance of the UNET when applied for other ESMs different to the one used for training is considerably worse, indicating poor generalization. For the linear method a similar deterioration is seen, so this limitation does not seem method specific but inherent to the task. For the second experiment, the emulators are trained in present and evaluated in future, under extrapolation. While averaged aspects such as the mean values are well simulated in future, significant biases (up to 5°C) appear when assessing warm extremes. These biases are larger by UNET than those produced by the linear method. This limitation suggests that, for variables such as temperature, with a marked signal of change and a strong linear relationship with predictors, simple linear methods might be more appropriate than the sophisticated deep learning techniques. | es_ES |
dc.language.iso | eng | es_ES |
dc.publisher | Royal Meteorological Society | es_ES |
dc.publisher | John Wiley & Sons | es_ES |
dc.rights | Licencia CC: Reconocimiento CC BY | es_ES |
dc.subject | Convolutional neural networks | es_ES |
dc.subject | Deep learning | es_ES |
dc.subject | Emulators | es_ES |
dc.subject | Evaluation | es_ES |
dc.subject | EURO-CORDEX | es_ES |
dc.subject | Pseudo-reality | es_ES |
dc.subject | Extrapolation | es_ES |
dc.subject | Statistical downscaling | es_ES |
dc.subject | Regional climate models | es_ES |
dc.title | On the limitations of deep learning for statistical downscaling of climate change projections : the transferability and the extrapolation issues | es_ES |
dc.type | info:eu-repo/semantics/article | es_ES |
dc.relation.publisherversion | https://doi.org/10.1002/asl.1195 | es_ES |
dc.rights.accessRights | info:eu-repo/semantics/openAccess | es_ES |
Colecciones: | Artículos científicos 2023-2026 |
Ficheros en este ítem:
Fichero | Descripción | Tamaño | Formato | ||
---|---|---|---|---|---|
![]() | ASL_Hernanz_2023.pdf | 2,44 MB | Adobe PDF | ![]() Visualizar/Abrir |
Los ítems de Arcimis están protegidos por una Licencia Creative Commons, salvo que se indique lo contrario.
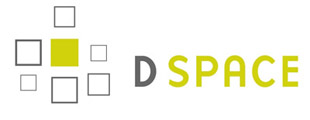