Por favor, use este identificador para citar o enlazar este ítem:
http://hdl.handle.net/20.500.11765/16544
Empirical methods to determine surface air temperature from satellite-retrieved data
Registro completo de metadatos
Campo DC | Valor | Lengua/Idioma |
---|---|---|
dc.contributor.author | Vedrí, Joan | es_ES |
dc.contributor.author | Niclòs, Raquel | es_ES |
dc.contributor.author | Pérez-Planells, Lluís | es_ES |
dc.contributor.author | Valor, Enric | es_ES |
dc.contributor.author | Luna Rico, Yolanda | es_ES |
dc.contributor.author | Estrela Navarro, María José | es_ES |
dc.date.accessioned | 2025-02-06T15:21:11Z | - |
dc.date.available | 2025-02-06T15:21:11Z | - |
dc.date.issued | 2025 | - |
dc.identifier.citation | International Journal of Applied Earth Observation and Geoinformation. 2025, 136, 104380 | es_ES |
dc.identifier.issn | 0303-2434 | - |
dc.identifier.issn | 1872-826X | - |
dc.identifier.uri | http://hdl.handle.net/20.500.11765/16544 | - |
dc.description.abstract | Surface air temperature (SAT) is an essential climate variable (ECV). Models based on remote sensing data allow us to study SAT, without the need for a large network of meteorological stations. Therefore, it allows monitoring the climate in remote and extensive areas. Niclos et al. (2014) proposed parametric equations for the SAT retrieval over the Spanish Mediterranean basins. In this study, we evaluated those equations, but in a larger area and period of study. In addition, we proposed several linear regression models and nonlinear models based on decision tree methods, non-parametric methods and neuronal networks. These models relate SAT to land surface temperature, vegetation indexes and albedo from MODIS data. Moreover, meteorological reanalysis data, from ERA5-Land database, and geographical parameters were used. The accuracy of each model was evaluated against data from meteorological stations operated by AEMET in the Spanish Mediterranean basins, during the period 2021–2022. The equations of Niclos et al. (2014) obtained a robust root mean square error (RRMSE) of 3.1 K at daytime and 1.9 K at nighttime. For the linear regression models, the RRMSE decreased to 2.3 K (1.5 K) at daytime (nighttime). Finally, the nonlinear methods, in particular XGBoost model, showed an RRMSE of 1.5 K for daytime and 1.0 K at nighttime. Therefore, the comparison between methods showed that nonlinear models, in particular those based on decision tree methods, offered the best results in SAT retrieval in our study. | es_ES |
dc.description.sponsorship | The study was conducted within the framework of the project Tool4Extreme PID2020-118797RBI00 funded by Ministerio de Ciencia e Innovacion and Agencia Estatal de Investigacion (MCIN/AEI/10.130 39/501100011033). Also, we thanks the project PROMETEO/2021/016 funded by Conselleria de Educacion, Universidades y Empleo, Generalitat Valenciana, Spain. | es_ES |
dc.language.iso | eng | es_ES |
dc.publisher | Elsevier | es_ES |
dc.rights | Licencia CC: Reconocimiento-NoComercial CC BY-NC | es_ES |
dc.subject | Surface air temperature | es_ES |
dc.subject | Land surface temperature | es_ES |
dc.subject | Climate change | es_ES |
dc.subject | Machine learning | es_ES |
dc.subject | Essential climate variable | es_ES |
dc.title | Empirical methods to determine surface air temperature from satellite-retrieved data | es_ES |
dc.type | info:eu-repo/semantics/article | es_ES |
dc.relation.publisherversion | https://doi.org/10.1016/j.jag.2025.104380 | es_ES |
dc.rights.accessRights | info:eu-repo/semantics/openAccess | es_ES |
Colecciones: | Artículos científicos 2023-2026 |

Ficheros en este ítem:
Fichero | Descripción | Tamaño | Formato | ||
---|---|---|---|---|---|
![]() | IJAEOG_Vedri_2025.pdf | 5,47 MB | Adobe PDF | ![]() Visualizar/Abrir |
Los ítems de Arcimis están protegidos por una Licencia Creative Commons, salvo que se indique lo contrario.
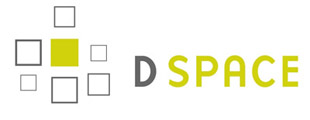