Por favor, use este identificador para citar o enlazar este ítem:
http://hdl.handle.net/20.500.11765/11578
The VALUE perfect predictor experiment: evaluation of temporal variability
Registro completo de metadatos
Campo DC | Valor | Lengua/Idioma |
---|---|---|
dc.contributor.author | Maraun, Douglas | es_ES |
dc.contributor.author | Huth, Radan | es_ES |
dc.contributor.author | Gutiérrez Llorente, José Manuel | es_ES |
dc.contributor.author | San-Martín, Daniel | es_ES |
dc.contributor.author | Dubrovsky, Martin | es_ES |
dc.contributor.author | Fischer, Andreas M. | es_ES |
dc.contributor.author | Hertig, Elke | es_ES |
dc.contributor.author | Soares, Pedro M. M. | es_ES |
dc.contributor.author | Bartholy, Judit | es_ES |
dc.contributor.author | Pongracz, Rita | es_ES |
dc.contributor.author | Widmann, Martin | es_ES |
dc.contributor.author | Casado Calle, María Jesús | es_ES |
dc.contributor.author | Ramos Calzado, Petra | es_ES |
dc.contributor.author | Bedia, Joaquín | es_ES |
dc.date.accessioned | 2020-04-06T11:32:45Z | - |
dc.date.available | 2020-04-06T11:32:45Z | - |
dc.date.issued | 2019 | - |
dc.identifier.citation | International Journal of Climatology. 2019, 39(9), p. 3786-3818 | es_ES |
dc.identifier.issn | 0899-8418 | - |
dc.identifier.issn | 1097-0088 | - |
dc.identifier.uri | http://hdl.handle.net/20.500.11765/11578 | - |
dc.description.abstract | Temporal variability is an important feature of climate, comprising systematic vari-ations such as the annual cycle, as well as residual temporal variations such asshort-term variations, spells and variability from interannual to long-term trends.The EU-COST Action VALUE developed a comprehensive framework to evaluatedownscaling methods. Here we present the evaluation of the perfect predictorexperiment for temporal variability. Overall, the behaviour of the differentapproaches turned out to be as expected from their structure and implementation.The chosen regional climate model adds value to reanalysis data for most consid-ered aspects, for all seasons and for both temperature and precipitation. Bias cor-rection methods do not directly modify temporal variability apart from the annualcycle. However, wet day corrections substantially improve transition probabilitiesand spell length distributions, whereas interannual variability is in some cases dete-riorated by quantile mapping. The performance of perfect prognosis (PP) statisticaldownscaling methods varies strongly from aspect to aspect and method to method,and depends strongly on the predictor choice. Unconditional weather generatorstend to perform well for the aspects they have been calibrated for, but underrepre-sent long spells and interannual variability. Long-term temperature trends of thedriving model are essentially unchanged by bias correction methods. If precipita-tion trends are not well simulated by the driving model, bias correction furtherdeteriorates these trends. The performance of PP methods to simulate trendsdepends strongly on the chosen predictors. | es_ES |
dc.description.sponsorship | VALUE has been funded as EU COST Action ES1102. | es_ES |
dc.language.iso | eng | es_ES |
dc.publisher | Wiley | es_ES |
dc.publisher | Royal Meteorological Society | es_ES |
dc.subject | Downscaling | es_ES |
dc.subject | Evaluation | es_ES |
dc.subject | Interannual variability | es_ES |
dc.subject | Long-term trends | es_ES |
dc.subject | Regionalclimate | es_ES |
dc.subject | Spells | es_ES |
dc.subject | Temporal variability | es_ES |
dc.subject | Validation | es_ES |
dc.title | The VALUE perfect predictor experiment: evaluation of temporal variability | es_ES |
dc.type | info:eu-repo/semantics/article | es_ES |
dc.relation.publisherversion | https://dx.doi.org/10.1002/joc.5222 | es_ES |
dc.rights.accessRights | info:eu-repo/semantics/openAccess | es_ES |
Colecciones: | Artículos científicos 2019-2022 |

Ficheros en este ítem:
Fichero | Descripción | Tamaño | Formato | ||
---|---|---|---|---|---|
![]() | 2017_Maraun_IJOC.pdf | 1,46 MB | Adobe PDF | ![]() Visualizar/Abrir |
Los ítems de Arcimis están protegidos por una Licencia Creative Commons, salvo que se indique lo contrario.
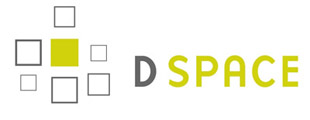