Por favor, use este identificador para citar o enlazar este ítem:
http://hdl.handle.net/20.500.11765/11746
Homogenizing GPS integrated water vapor time series: benchmarking break detection methods on synthetic data sets
Título : | Homogenizing GPS integrated water vapor time series: benchmarking break detection methods on synthetic data sets |
Autor : | Van Malderen, Roeland; Pottiaux, Eric; Klos, Anna; Domonkos, Peter; Elias, Michal; Ning, Tong; Bock, Olivier; Guijarro Pastor, José Antonio
![]() ![]() ![]() |
Palabras clave : | Water vapor; Global Positioning System; Break detection methods |
Fecha de publicación : | 2020 |
Editor: | American Geophysical Union |
Citación : | Earth and Space Science. 2020, 7(5) |
Versión del editor: | https://doi.org/10.1029/2020EA001121 |
Resumen : | We assess the performance of different break detection methods on three sets of benchmark data sets, each consisting of 120 daily time series of integrated water vapor differences. These differences are generated from the Global Positioning System (GPS) measurements at 120 sites worldwide, and the numerical weather prediction reanalysis (ERA‐Interim) integrated wáter vapor output, which serves as the reference series here. The benchmark includes homogeneous and inhomogeneous sections with added nonclimatic shifts (breaks) in the latter. Three different variants of the benchmark time series are produced, with increasing complexity, by adding autoregressive noise of the first order to the white noise model and the periodic behavior and consecutively by adding gaps and allowing nonclimatic trends. The purpose of this “complex experiment” is to examine the performance of break detection methods in a more realistic case when the reference series are not homogeneous. We evaluate the performance of break detection methods with skill scores, centered root mean square errors (CRMSE), and trend differences relative to the trends of the homogeneous series. We found that most methods underestimate the number of breaks and have a significant number of false detections. Despite this, the degree of CRMSE reduction is significant (roughly between 40% and 80%) in the easy to moderate experiments, with the ratio of trend bias reduction is even exceeding the 90% of the raw data error. For the complex experiment, the improvement ranges between 15% and 35% with respect to the raw data, both in terms of RMSE and trend estimations. |
Patrocinador: | The research has been undertaken in the framework of the European COST Action ES1206 GNSS4SWEC (GNSS for Severe Weather and Climate monitoring; http://www.cost.eu/COST_Actions/essem/ES1206), which also funded two dedicated workshops on this activity. |
URI : | http://hdl.handle.net/20.500.11765/11746 |
ISSN : | 2333-5084 |
Colecciones: | Artículos científicos 2019-2022 |
Ficheros en este ítem:
Fichero | Descripción | Tamaño | Formato | ||
---|---|---|---|---|---|
![]() | 2020EA001121.pdf | 6,72 MB | Adobe PDF | ![]() Visualizar/Abrir |
Los ítems de Arcimis están protegidos por una Licencia Creative Commons, salvo que se indique lo contrario.
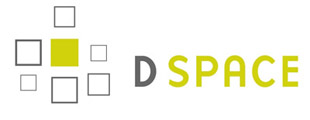