Por favor, use este identificador para citar o enlazar este ítem:
http://hdl.handle.net/20.500.11765/13613
A critical view on the suitability of machine learning techniques to downscale climate change projections : illustration for temperature with a toy experiment
Registro completo de metadatos
Campo DC | Valor | Lengua/Idioma |
---|---|---|
dc.contributor.author | Hernanz Lázaro, Alfonso | es_ES |
dc.contributor.author | García Valero, Juan Andrés | es_ES |
dc.contributor.author | Domínguez Alonso, Marta | es_ES |
dc.contributor.author | Rodríguez Camino, Ernesto | es_ES |
dc.date.accessioned | 2022-05-11T09:06:16Z | - |
dc.date.available | 2022-05-11T09:06:16Z | - |
dc.date.issued | 2022 | - |
dc.identifier.citation | Atmospheric Science Letters. 2022, e1087 | es_ES |
dc.identifier.issn | 1530-261X | - |
dc.identifier.uri | http://hdl.handle.net/20.500.11765/13613 | - |
dc.description.abstract | Machine learning is a growing field of research with many applications. It provides a series of techniques able to solve complex nonlinear problems, and that has promoted their application for statistical downscaling. Intercomparison exercises with other classical methods have so far shown promising results. Nevertheless, many evaluation studies of statistical downscaling methods neglect the analysis of their extrapolation capability. In this study, we aim to make a wakeup call to the community about the potential risks of using machine learning for statistical downscaling of climate change projections. We present a set of three toy experiments, applying three commonly used machine learning algorithms, two different implementations of artificial neural networks and a support vector machine, to downscale daily maximum temperature, and comparing them with the classical multiple linear regression. We have tested the four methods in and out of their calibration range, and have found how the three machine learning techniques can perform poorly under extrapolation. Additionally, we have analysed the impact of this extrapolation issue depending on the degree of overlapping between the training and testing datasets, and we have found very different sensitivities for each method and specific implementation. | es_ES |
dc.language.iso | eng | es_ES |
dc.publisher | Royal Meteorological Society | es_ES |
dc.publisher | Wiley | es_ES |
dc.rights | Licencia CC: Reconocimiento CC BY | es_ES |
dc.subject | Climate projections | es_ES |
dc.subject | Evaluation | es_ES |
dc.subject | Extrapolation | es_ES |
dc.subject | Neural networks | es_ES |
dc.subject | Machine learning | es_ES |
dc.subject | Statistical downscaling | es_ES |
dc.title | A critical view on the suitability of machine learning techniques to downscale climate change projections : illustration for temperature with a toy experiment | es_ES |
dc.type | info:eu-repo/semantics/article | es_ES |
dc.relation.publisherversion | https://doi.org/10.1002/asl.1087 | es_ES |
dc.rights.accessRights | info:eu-repo/semantics/openAccess | es_ES |
Colecciones: | Artículos científicos 2019-2022 |
Ficheros en este ítem:
Fichero | Descripción | Tamaño | Formato | ||
---|---|---|---|---|---|
![]() | ASL2022_Hernanz.pdf | 1,59 MB | Adobe PDF | ![]() Visualizar/Abrir |
Los ítems de Arcimis están protegidos por una Licencia Creative Commons, salvo que se indique lo contrario.
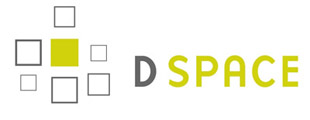