Por favor, use este identificador para citar o enlazar este ítem:
http://hdl.handle.net/20.500.11765/14305
Estimation of NO2 emission strengths over Riyadh and Madrid from space from a combination of wind-assigned anomalies and machine learning technique [Discussion paper]
Registro completo de metadatos
Campo DC | Valor | Lengua/Idioma |
---|---|---|
dc.contributor.author | Tu, Qiansi | es_ES |
dc.contributor.author | Hase, Frank | es_ES |
dc.contributor.author | Chen, Zihan | es_ES |
dc.contributor.author | Schneider, Matthias | es_ES |
dc.contributor.author | García Rodríguez, Omaira Elena | es_ES |
dc.contributor.author | Khosrawi, Farahnaz | es_ES |
dc.contributor.author | Blumenstock, Thomas | es_ES |
dc.contributor.author | Liu, Fang | es_ES |
dc.contributor.author | Qin, Kai | es_ES |
dc.contributor.author | Lin, Song | es_ES |
dc.contributor.author | Jiang, Hongyan | es_ES |
dc.contributor.author | Fang, Dianjun | es_ES |
dc.date.accessioned | 2023-01-13T10:46:24Z | - |
dc.date.available | 2023-01-13T10:46:24Z | - |
dc.date.issued | 2022 | - |
dc.identifier.citation | Atmospheric Measurement Techniques Discussions [Preprint]. 2022 | es_ES |
dc.identifier.issn | 1867-1381 | - |
dc.identifier.issn | 1867-8548 | - |
dc.identifier.uri | http://hdl.handle.net/20.500.11765/14305 | - |
dc.description.abstract | Nitrogen dioxide (NO2) air pollution provides valuable information for quantifying NOx emissions and exposures. This study presents a comprehensive method to estimate average tropospheric NO2 emission strengths derived from three-year (April 2018 – March 2021) TROPOMI observations by combining a wind-assigned anomaly approach and a Machine Learning (ML) method, the so-called Gradient Descent. This combined approach is firstly applied to the Saudi Arabian capital city Riyadh, as a test site, and yields a total emission rate of 1.04×1026 molec./s. The ML-trained anomalies fit very well with the wind-assigned anomalies with an R2 value of 1.0 and a slope of 0.99. Hotspots of NO2 emissions are apparent at several sites where the cement plant and power plants are located and over areas along the highways. Using the same approach, an emission rate of 1.80×1025 molec./s is estimated in the Madrid metropolitan area, Spain. Both the estimate and spatial pattern are comparable to the CAMS inventory. | es_ES |
dc.description.sponsorship | We also acknowledge the project of Joint R&D and Talents Program funded by the Qingdao Sino-German Institute of Intelligent Technologies (kh0100020213319) and the project of Transnational Interoperability Rules and Solution Patterns in Collaborative Production Networks based on IDS and GAIA-X funded by Ministry of Science and Technology, PRC (SQ2021YFE010470). | es_ES |
dc.language.iso | eng | es_ES |
dc.publisher | European Geosciences Union | es_ES |
dc.publisher | Copernicus Publications | es_ES |
dc.rights | Licencia CC: Reconocimiento CC BY | es_ES |
dc.subject | Nitrogen dioxide | es_ES |
dc.subject | Air pollution | es_ES |
dc.subject | Wind-assigned anomalies | es_ES |
dc.subject | Machine learning technique | es_ES |
dc.title | Estimation of NO2 emission strengths over Riyadh and Madrid from space from a combination of wind-assigned anomalies and machine learning technique [Discussion paper] | es_ES |
dc.type | info:eu-repo/semantics/preprint | es_ES |
dc.relation.publisherversion | https://doi.org/10.5194/amt-2022-176 | es_ES |
dc.rights.accessRights | info:eu-repo/semantics/openAccess | es_ES |
Colecciones: | Artículos científicos 2019-2022 |
Ficheros en este ítem:
Fichero | Descripción | Tamaño | Formato | ||
---|---|---|---|---|---|
![]() | AMTD_Tu_2022.pdf | 7,85 MB | Adobe PDF | ![]() Visualizar/Abrir |
Los ítems de Arcimis están protegidos por una Licencia Creative Commons, salvo que se indique lo contrario.
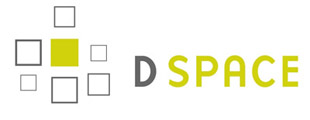