Por favor, use este identificador para citar o enlazar este ítem:
http://hdl.handle.net/20.500.11765/14466
Estimation of NO2 emission strengths over Riyadh and Madrid from space from a combination of wind-assigned anomalies and a machine learning technique
Título : | Estimation of NO2 emission strengths over Riyadh and Madrid from space from a combination of wind-assigned anomalies and a machine learning technique |
Autor : | Tu, Qiansi; Hase, Frank; Chen, Zihan; Schneider, Matthias
![]() ![]() ![]() ![]() |
Palabras clave : | Nitrogen dioxide; Air pollution; Wind-assigned anomalies; Machine learning technique |
Fecha de publicación : | 2023 |
Editor: | European Geosciences Union; Copernicus Publications |
Citación : | Atmospheric Measurement Techniques. 2023, 16(8), p. 2237–2262 |
Versión del editor: | https://doi.org/10.5194/amt-16-2237-2023 |
Resumen : | Nitrogen dioxide (NO2) air pollution provides valuable information for quantifying NOx (NOx = NO + NO2) emissions and exposures. This study presents a comprehensive method to estimate average tropospheric NO2 emission strengths derived from 4-year (May 2018–June 2022) TROPOspheric Monitoring Instrument (TROPOMI) observations by combining a wind-assigned anomaly approach and a machine learning (ML) method, the so-called gradient descent algorithm. This combined approach is firstly applied to the Saudi Arabian capital city of Riyadh, as a test site, and yields a total emission rate of 1.09×1026 molec. s−1. The ML-trained anomalies fit very well with the wind-assigned anomalies, with an R2 value of 1.0 and a slope of 0.99. Hotspots of NO2 emissions are apparent at several sites: over a cement plant and power plants as well as over areas along highways. Using the same approach, an emission rate of 1.99×1025 molec. s−1 is estimated in the Madrid metropolitan area, Spain. Both the estimate and spatial pattern are comparable with the Copernicus Atmosphere Monitoring Service (CAMS) inventory. |
Patrocinador: | We wish to acknowledge the Joint R&D and Talents Program project, funded by the Qingdao Sino-German Institute of Intelligent Technologies (grant no. kh0100020213319); the Deutsche Forschungsgemeinschaft; and the Open Access Publishing Fund of the Karlsruhe Institute of Technology for their support. |
URI : | http://hdl.handle.net/20.500.11765/14466 |
ISSN : | 1867-1381 1867-8548 |
Colecciones: | Artículos científicos 2023-2026 |
Ficheros en este ítem:
Fichero | Descripción | Tamaño | Formato | ||
---|---|---|---|---|---|
![]() | amt-16-2237-2023.pdf | 2,03 MB | Adobe PDF | ![]() Visualizar/Abrir |
Los ítems de Arcimis están protegidos por una Licencia Creative Commons, salvo que se indique lo contrario.
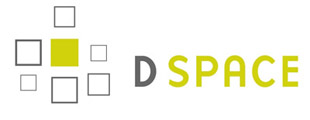