Por favor, use este identificador para citar o enlazar este ítem:
http://hdl.handle.net/20.500.11765/14865
Event selection for dynamical downscaling: a neural network approach for physically-constrained precipitation events
Registro completo de metadatos
Campo DC | Valor | Lengua/Idioma |
---|---|---|
dc.contributor.author | Gómez Navarro, Juan José | es_ES |
dc.contributor.author | Raible, Christoph Cornelius | es_ES |
dc.contributor.author | García Valero, Juan Andrés | es_ES |
dc.contributor.author | Messmer, Martina | es_ES |
dc.contributor.author | Montávez Gómez, Juan Pedro | es_ES |
dc.contributor.author | Martius, Olivia | es_ES |
dc.date.accessioned | 2023-08-02T07:59:17Z | - |
dc.date.available | 2023-08-02T07:59:17Z | - |
dc.date.issued | 2022 | - |
dc.identifier.citation | Climate Dynamics. 2022, 58, p. 2863–2879 | es_ES |
dc.identifier.issn | 0930-7575 | - |
dc.identifier.issn | 1432-0894 | - |
dc.identifier.uri | http://hdl.handle.net/20.500.11765/14865 | - |
dc.description.abstract | This study presents a new dynamical downscaling strategy for extreme events. It is based on a combination of statistical downscaling of coarsely resolved global model simulations and dynamical downscaling of specific extreme events constrained by the statistical downscaling part. The method is applied to precipitation extremes over the upper Aare catchment, an area in Switzerland which is characterized by complex terrain. The statistical downscaling part consists of an Artificial Neural Network (ANN) framework trained in a reference period. Thereby, dynamically downscaled precipitation over the target area serve as predictands and large-scale variables, received from the global model simulation, as predictors. Applying the ANN to long term global simulations produces a precipitation series that acts as a surrogate of the dynamically downscaled precipitation for a longer climate period, and therefore are used in the selection of events. These events are then dynamically downscaled with a regional climate model to 2 km. The results show that this strategy is suitable to constraint extreme precipitation events, although some limitations remain, e.g., the method has lower efficiency in identifying extreme events in summer and the sensitivity of extreme events to climate change is underestimated. | es_ES |
dc.language.iso | eng | es_ES |
dc.publisher | Springer | es_ES |
dc.subject | Dynamical downscaling | es_ES |
dc.subject | Extreme events | es_ES |
dc.subject | Statistical downscaling | es_ES |
dc.subject | Precipitation extremes | es_ES |
dc.title | Event selection for dynamical downscaling: a neural network approach for physically-constrained precipitation events | es_ES |
dc.type | info:eu-repo/semantics/preprint | es_ES |
dc.relation.publisherversion | https://doi.org/10.1007/s00382-019-04818-w | es_ES |
dc.rights.accessRights | info:eu-repo/semantics/openAccess | es_ES |
Colecciones: | Artículos científicos 2019-2022 |
Ficheros en este ítem:
Fichero | Descripción | Tamaño | Formato | ||
---|---|---|---|---|---|
![]() | CD_Gomez_2019.pdf | 1,19 MB | Adobe PDF | ![]() Visualizar/Abrir |
Los ítems de Arcimis están protegidos por una Licencia Creative Commons, salvo que se indique lo contrario.
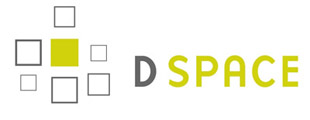