Por favor, use este identificador para citar o enlazar este ítem:
http://hdl.handle.net/20.500.11765/15347
Thunderstorm prediction during pre-tactical air-traffic-flow management using convolutional neural networks
Registro completo de metadatos
Campo DC | Valor | Lengua/Idioma |
---|---|---|
dc.contributor.author | Jardines, Aniel | es_ES |
dc.contributor.author | Eivazi, Hamidreza | es_ES |
dc.contributor.author | Zea, Elias | es_ES |
dc.contributor.author | García-Heras, Javier | es_ES |
dc.contributor.author | Simarro Grande, Juan Pablo | es_ES |
dc.contributor.author | Otero, Evelyn | es_ES |
dc.contributor.author | Soler, Manuel | es_ES |
dc.contributor.author | Vinuesa, Ricardo | es_ES |
dc.date.accessioned | 2024-01-09T07:47:06Z | - |
dc.date.available | 2024-01-09T07:47:06Z | - |
dc.date.issued | 2024 | - |
dc.identifier.citation | Expert Systems with Applications. 2024, 241, 122466 | es_ES |
dc.identifier.issn | 0957-4174 | - |
dc.identifier.uri | http://hdl.handle.net/20.500.11765/15347 | - |
dc.description.abstract | Thunderstorms can be a large source of disruption for European air-traffic management causing a chaotic state of operation within the airspace system. In current practice, air-traffic managers are provided with imprecise forecasts which limit their ability to plan strategically. As a result, weather mitigation is performed using tactical measures with a time horizon of three hours. Increasing the lead time of thunderstorm predictions to the day before operations could help air-traffic managers plan around weather and improve the efficiency of air-traffic-management operations. Emerging techniques based on machine learning have provided promising results, partly attributed to reduced human bias and improved capacity in predicting thunderstorms purely from numerical weather prediction data. In this paper, we expand on our previous work on thunderstorm forecasting, by applying convolutional neural networks (CNNs) to exploit the spatial characteristics embedded in the weather data. The learning task of predicting convection is formulated as a binary-classification problem based on satellite data. The performance of multiple CNN-based architectures, including a fully-convolutional neural network (FCN), a CNN-based encoder–decoder, a U-Net, and a pyramid-scene parsing network (PSPNet) are compared against a multi-layer-perceptron (MLP) network. Our work indicates that CNN-based architectures improve the performance of point-prediction models, with a fully-convolutional neural-network architecture having the best performance. Results show that CNN-based architectures can be used to increase the prediction lead time of thunderstorms. Lastly, a case study illustrating the applications of convection-prediction models in an air-traffic-management setting is presented. | es_ES |
dc.description.sponsorship | The project has also received funding from the SESAR Joint Undertaking within the framework of the European Union’s Horizon 2020 research and innovation programme under grant agreement No 891965. | es_ES |
dc.language.iso | eng | es_ES |
dc.publisher | Elsevier | es_ES |
dc.rights | Licencia CC: Reconocimiento–NoComercial–SinObraDerivada CC BY-NC-ND | es_ES |
dc.subject | Thunderstorms | es_ES |
dc.subject | Air-traffic management | es_ES |
dc.subject | Weather data | es_ES |
dc.subject | Numerical weather prediction | es_ES |
dc.subject | Satellite images | es_ES |
dc.subject | Machine learning | es_ES |
dc.subject | Convolutional neural network | es_ES |
dc.title | Thunderstorm prediction during pre-tactical air-traffic-flow management using convolutional neural networks | es_ES |
dc.type | info:eu-repo/semantics/article | es_ES |
dc.relation.publisherversion | https://doi.org/10.1016/j.eswa.2023.122466 | es_ES |
dc.rights.accessRights | info:eu-repo/semantics/openAccess | es_ES |
Colecciones: | Artículos científicos 2023-2026 |

Ficheros en este ítem:
Fichero | Descripción | Tamaño | Formato | ||
---|---|---|---|---|---|
![]() | ESA_Jardines_2023_com... | 875,47 kB | Adobe PDF | ![]() Visualizar/Abrir |
Los ítems de Arcimis están protegidos por una Licencia Creative Commons, salvo que se indique lo contrario.
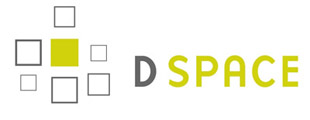