Por favor, use este identificador para citar o enlazar este ítem:
http://hdl.handle.net/20.500.11765/16119
A neural network to retrieve cloud cover from all-sky cameras: A case of study over Antarctica
Título : | A neural network to retrieve cloud cover from all-sky cameras: A case of study over Antarctica |
Autor : | González-Fernández, Daniel; Román, Roberto; Antuña-Sánchez, Juan Carlos; Cachorro, Victoria E.; Copes, Gustavo; Herrero-Anta, Sara; Herrero del Barrio, Celia; Barreto Velasco, África
![]() ![]() ![]() ![]() ![]() |
Palabras clave : | AI; All-sky camera; Antarctic; Convolutional neural network; Cloud cover; Image identification |
Fecha de publicación : | 2024 |
Editor: | Wiley; Royal Meteorological Society |
Citación : | Quarterly Journal of the Royal Meteorological Society. 2024, Early View |
Versión del editor: | https://doi.org/10.1002/qj.4834 |
Resumen : | We present a new model based on a convolutional neural network (CNN) to predict daytime cloud cover (CC) from sky images captured by all-sky cameras, which is called CNN-CC. A total of 49,016 daytime sky images, recorded at different Spanish locations (Valladolid, La Palma, and Izaña) from two different all-sky camera types, are manually classified into different CC (oktas) values by trained researchers. Subsequently, the images are randomly split into a training set and a test set to validate the model. The CC values predicted by the CNN-CC model are compared with the observations made by trained people on the test set, which serve as reference. |
Patrocinador: | The research has been supported by the Ministeriode Ciencia e Innovación (MICINN), with Grant no.PID2021-127588OB-I00, and the Junta of Castilla y León (JCyL) with Grant no. VA227P20. This work ispart of the project TED2021-131211B-I00 funded byMCIN/AEI/10.13039/501100011033 and the EuropeanUnion, “NextGenerationEU”/PRTR. |
URI : | http://hdl.handle.net/20.500.11765/16119 |
ISSN : | 0035-9009 1477-870X |
Colecciones: | Artículos científicos 2023-2026 |
Ficheros en este ítem:
Fichero | Descripción | Tamaño | Formato | ||
---|---|---|---|---|---|
![]() | QJRMS_Gonzalez_2024.pdf | 9,72 MB | Adobe PDF | ![]() Visualizar/Abrir |
Los ítems de Arcimis están protegidos por una Licencia Creative Commons, salvo que se indique lo contrario.
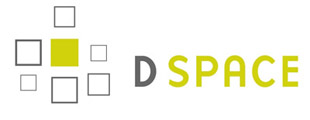