Por favor, use este identificador para citar o enlazar este ítem:
http://hdl.handle.net/20.500.11765/10537
Statistical postprocessing of different variables for airports in Spain using machine learning
Título : | Statistical postprocessing of different variables for airports in Spain using machine learning |
Autor : | Quintero Plaza, David; García-Moya, José Antonio |
Palabras clave : | Airports; Machine learning; Calibration; Wind speed; Precipitation |
Fecha de publicación : | 2019 |
Editor: | Hindawi |
Citación : | Advances in Meteorology. 2019, p. 1-14 |
Versión del editor: | https://dx.doi.org/10.1155/2019/3181037 |
Resumen : | The results of a deterministic calibration for the nonhydrostatic convection-permitting LAM-EPS AEMET-γSREPS are shown. LAM-EPS AEMET-γSREPS is a multiboundary condition, multimodel ensemble forecast system developed for Spain. Machine learning tools are used to calibrate the members of the ensemble. Machine learning (hereafter ML) has been considerably successful in many problems, and recent research suggests that meteorology and climatology are not an exception. These machine learning tools range from classical statistical methods to contemporary successful and powerful methods such as kernels and neural networks. The calibration has been done for airports located in many regions of Spain, representing different climatic conditions. The variables to be calibrated are the 2-meter temperature, the 10-meter wind speed, and the precipitation in 24 hours. Classical statistical methods perform very well with the temperature and the wind speed; the precipitation is a subtler case: it seems there is not a general rule, and for each point, a decision has to be taken of what method (if any) improves the direct output of the model, but even recognizing this, a slight improvement can be shown with ML methods for the precipitation. |
Patrocinador: | The authors thank the Spanish weather service, AEMET, for its funding and support, both the headquarters office and the local office of the Canary Islands. |
URI : | http://hdl.handle.net/20.500.11765/10537 |
ISSN : | 1687-9309 |
Colecciones: | Artículos científicos 2019-2022 |
Ficheros en este ítem:
Fichero | Descripción | Tamaño | Formato | ||
---|---|---|---|---|---|
![]() | Quintero_Statistical_... | 4,13 MB | Adobe PDF | ![]() Visualizar/Abrir |
Los ítems de Arcimis están protegidos por una Licencia Creative Commons, salvo que se indique lo contrario.
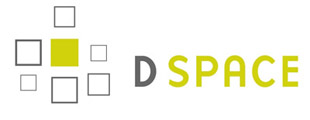