Por favor, use este identificador para citar o enlazar este ítem:
http://hdl.handle.net/20.500.11765/14706
Statistical Downscaling in the Tropics and Midlatitudes: A Comparative Assessment over Two Representative Regions
Registro completo de metadatos
Campo DC | Valor | Lengua/Idioma |
---|---|---|
dc.contributor.author | Hernanz Lázaro, Alfonso | es_ES |
dc.contributor.author | Correa, Carlos | es_ES |
dc.contributor.author | Domínguez Alonso, Marta | es_ES |
dc.contributor.author | Rodríguez Guisado, Esteban | es_ES |
dc.contributor.author | Rodríguez Camino, Ernesto | es_ES |
dc.date.accessioned | 2023-07-14T10:41:19Z | - |
dc.date.available | 2023-07-14T10:41:19Z | - |
dc.date.issued | 2023 | - |
dc.identifier.citation | Journal of Applied Meteorology and Climatology. 2023, 62(6), p. 737–753 | es_ES |
dc.identifier.issn | 1558-8424 | - |
dc.identifier.issn | 1558-8432 | - |
dc.identifier.uri | http://hdl.handle.net/20.500.11765/14706 | - |
dc.description.abstract | Statistical downscaling (SD) of climate change projections is a key piece for impact and adaptation studies due to its low computational expense compared to dynamical downscaling, which allows exploration of uncertainties through the generation of large ensembles. SD has been extensively evaluated and applied in the extratropics, but few examples exist in tropical regions. In this study, several state-of-the-art methods belonging to different families have been evaluated for maximum/minimum daily temperature and daily accumulated precipitation (both from the ERA5 at 0.258) in two regions with very different climates: Spain (midlatitudes) and Central America (tropics). Some key assumptions of SD have been tested: the strength of the predictor–predictand links, the skill of different approaches, and the extrapolation capability of each method. It has been found that relevant predictors are different in both regions, as is the behavior of statistical methods. For temperature, most methods perform significantly better in Spain than in Central America, where transfer function (TF) methods present important extrapolation problems, probably due to the low variability of the training sample (present climate). In both regions, model output statistics (MOS) methods have achieved the best results for temperature. In Central America, TF methods have achieved better results than MOS methods in the evaluation in the present climate, but they do not preserve trends in the future. For precipitation, MOS methods and the extreme gradient boost machine learning method have achieved the best results in both regions. In addition, it has been found that, although the use of humidity indices as predictors improves results for the downscaling of precipitation, future trends given by statistical methods are very sensitive to the use of one or another index. Three indices have been compared: relative humidity, specific humidity, and dewpoint depression. The use of the specific humidity has been found to lead to trends given by the downscaled projections that deviate seriously from those given by raw global climate models in both regions. | es_ES |
dc.language.iso | eng | es_ES |
dc.publisher | American Meteorological Society | es_ES |
dc.rights | Licencia CC: Reconocimiento CC BY | es_ES |
dc.subject | Extratropics | es_ES |
dc.subject | Tropics | es_ES |
dc.subject | Climate change | es_ES |
dc.subject | Machine learning | es_ES |
dc.subject | Downscaling | es_ES |
dc.title | Statistical Downscaling in the Tropics and Midlatitudes: A Comparative Assessment over Two Representative Regions | es_ES |
dc.type | info:eu-repo/semantics/article | es_ES |
dc.relation.publisherversion | https://doi.org/10.1175/JAMC-D-22-0164.1 | es_ES |
dc.rights.accessRights | info:eu-repo/semantics/openAccess | es_ES |
Colecciones: | Artículos científicos 2023-2026 |
Ficheros en este ítem:
Fichero | Descripción | Tamaño | Formato | ||
---|---|---|---|---|---|
![]() | JAMC-D-22-0164.1.pdf | 3,21 MB | Adobe PDF | ![]() Visualizar/Abrir | |
![]() | JAMC-D-22-0164.s1.pdf | 854,08 kB | Adobe PDF | ![]() Visualizar/Abrir |
Los ítems de Arcimis están protegidos por una Licencia Creative Commons, salvo que se indique lo contrario.
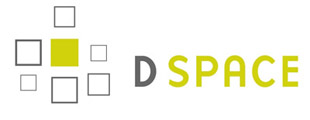